As industries worldwide confront the challenging task of minimizing costs associated with unexpected downtime, the predictive maintenance sector has emerged as a source of optimism. According to the most recent Predictive Maintenance and Asset Performance industry Report 2023-2028, the global predictive maintenance industry will reach $5.5 billion in 2022, an excellent 11% increase over the previous year. Furthermore, this market is expected to grow at a strong Compound Annual Growth Rate (CAGR) of 17% through 2028. These numbers highlight the growing importance of effectively predicting significant asset failures to reduce the startling median unexpected downtime costs, which frequently approach $100,000 per hour.
In the field of predictive maintenance, the report identified key features that shed light on the market’s development and possibilities.
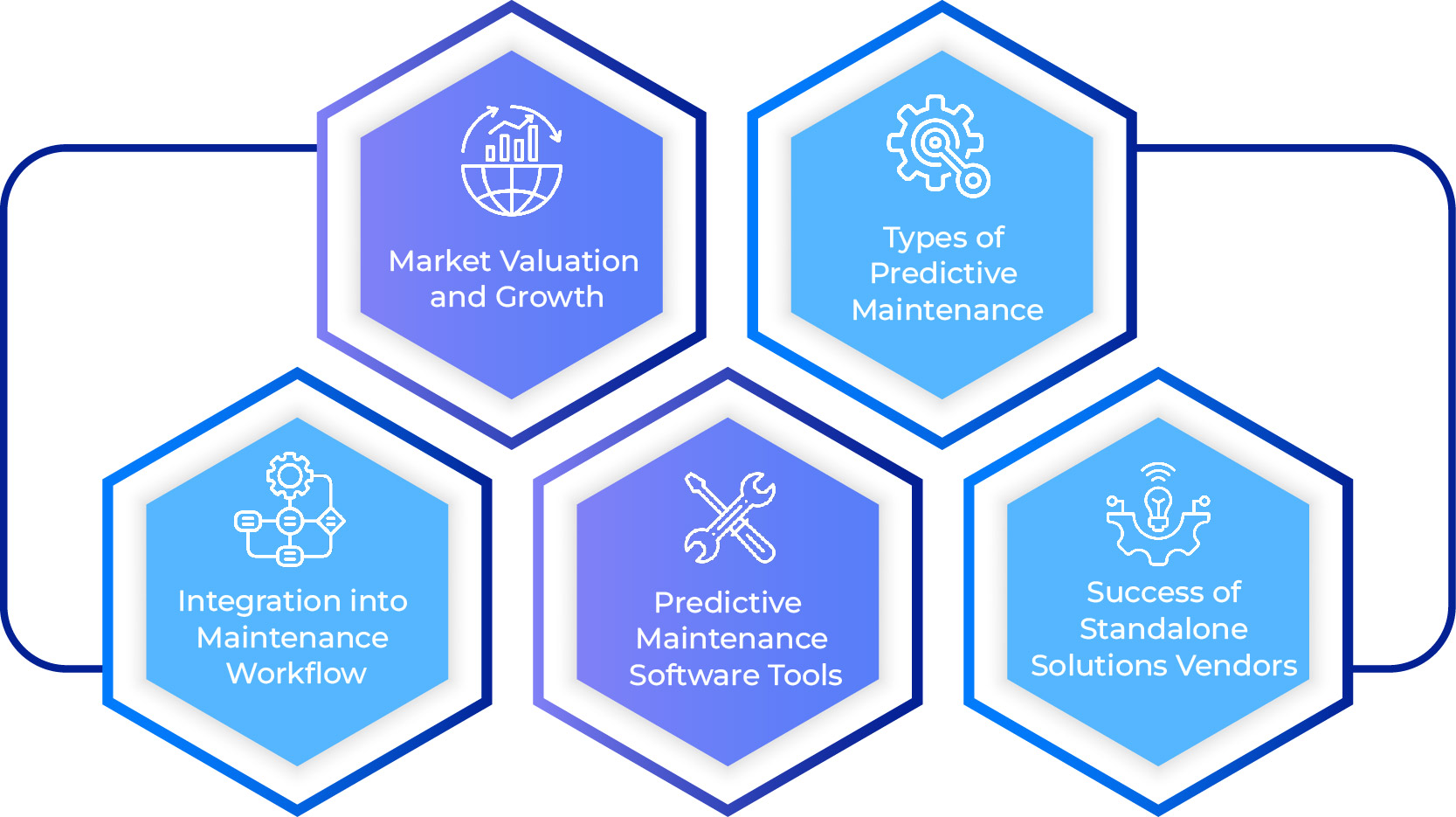
Market Valuation and Growth: The market’s valuation of $5.5 billion in 2022 firmly establishes it as a vital and expanding industry. This rising trend reflects the increasing need for predictive maintenance solutions across a wide range of sectors.
Types of Predictive Maintenance: The creation of three separate types of predictive maintenance, including indirect failure prediction, anomaly detection, and remaining usable life (RUL), reflects the various methodologies used to anticipate and prevent operational disturbances.
Predictive Maintenance Software Tools: The report identified six common qualities among predictive maintenance software tools, including data collecting, analytics, and status visualization. This emphasizes technological breakthroughs and functionality that are shaping the predictive maintenance landscape.
Integration into Maintenance Workflow: The seamless integration of predictive maintenance solutions into broader Asset Performance Management (APM) and Computerized Maintenance Management Systems (CMMS) represents a significant change toward more holistic and optimized maintenance tactics.
Success of Standalone Solutions Vendors: The success of independent sellers of predictive maintenance systems was an intriguing discovery. By focusing on certain industries or assets, these suppliers drive innovation and personalized solutions, which contribute considerably to market growth.
Despite the optimistic outlook for predictive maintenance, some obstacles remain, most notably the low accuracy of many solutions, which frequently falls below 50%. This has created significant challenges for maintenance organizations, eroding trust and preventing the seamless deployment of predictive maintenance solutions.
Despite these hurdles, organizations continue to be drawn to the prospect of significant cost reductions and increased operational efficiency. According to the report, predictive maintenance systems have helped firms save hundreds of thousands of dollars, emphasizing the real benefits and possible return on investment (ROI).
As decision-makers navigate this changing landscape, they must grasp the many types of predictive maintenance solutions, software features, and the possibilities for personalized, industry-specific services to make sound investment decisions for their assets.
Exploring Predictive Maintenance Types
As businesses seek efficient predictive maintenance solutions, it is critical to understand the many types of predictive maintenance and how they can improve operational efficiency and reduce costs.
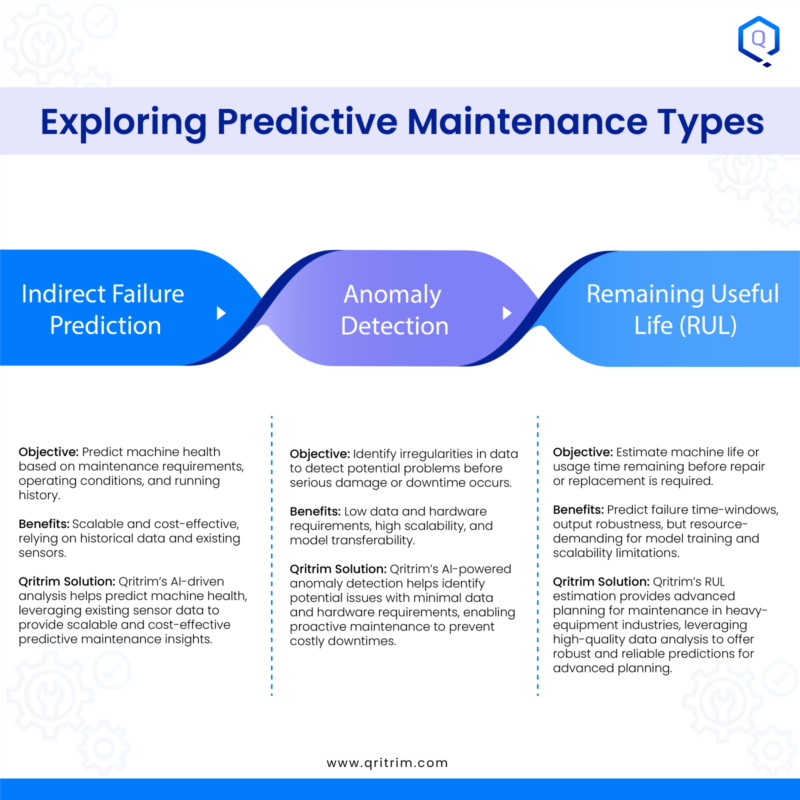
Indirect Failure Prediction
Predict machine health based on maintenance needs, operating circumstances, and use history.
Benefits: Scalable and cost-effective, utilizing past data and current sensors.
Qritrim Solution: Qritrim’s AI-driven analysis predicts machine health by using current sensor data to give scalable and cost-effective predictive maintenance insights.
Anomaly Detection
Identify data abnormalities in order to discover possible problems before they cause major harm or downtime.
Benefits: include low data and hardware needs, excellent scalability, and model transferability.
Qritrim Solution: Qritrim’s AI-powered anomaly detection identifies possible faults with little data and hardware needs, allowing for preventive maintenance to avoid costly downtime.
Remaining Useful Life (RUL)
The goal is to estimate the amount of time the machine will last before it needs to be repaired or replaced
Benefits: Predict failure time frames, output robustness, however model training requires a lot of resources and has scalability limits.
Qritrim Solutions: Qritrim’s RUL estimation enables advanced maintenance planning in the heavy-equipment industry by utilizing high-quality data analysis to give strong and dependable projections.
Qritrim’s Contribution to Predictive Maintenance Solutions
Qritrim provides cutting-edge AI solutions for reducing downtime and optimizing operations with predictive maintenance. Qritrim’s products use advanced AI-driven analysis to give scalable, cost-effective insights into machine health and potential issues. With an emphasis on low data and hardware needs, Qritrim’s AI-powered anomaly detection enables proactive maintenance to avoid costly downtime. Furthermore, Qritrim’s RUL estimation enables advanced maintenance planning in the heavy-equipment industry, providing strong and reliable projections for optimal maintenance methods.
To summarize, the many types of predictive maintenance, together with technical improvements and an increased emphasis on accuracy, indicate a considerable move towards more proactive and efficient maintenance practices. These advancements hold the prospect of significant cost reductions and increased operational resilience for firms in a variety of industries.